The overlooked GenAI use case
Job post data reveals what companies plan to do with GenAI. The biggest use case is cleaning, processing, and analyzing data.
A recent, widely circulated blog post by Sequoia Partner David Cahn asks what he refers to as the $600B question about AI: in the future, how much AI infrastructure will be needed? A question at least equally interesting is: what will all that infrastructure be used for?
Companies talk about GenAI in their earnings. But it turns out the more interesting trail of breadcrumbs is in their job posts. I used data from my newco, Sumble, to understand where GenAI is being explored in the enterprise today.
I looked at GenAI projects mentioned in global job posts over the 12 months to October 2024 and mapped those projects to known business functions. (See a full feed of GenAI projects companies are tackling on Sumble.) I then compared the relative interest level in different use cases with the relative investment level by the VC community (investment data from Pitchbook).
The results are revealing: relative to the current interest level in the enterprise, GenAI for data analytics and decision support is receiving limited attention. In this category, I include cleaning, processing and analyzing data to answer business questions.
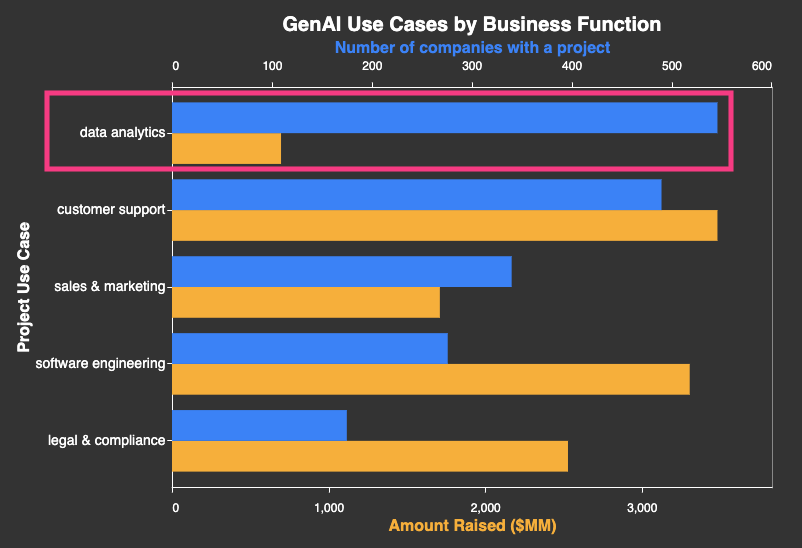
In the other major categories, there are many well-funded startups: customer support (Sierra, Kore.ai); software engineering (Poolside, Magic.dev); sales and marketing (Gong, Jasper); and legal and compliance (Harvey). By contrast, there’s been limited focus on GenAI for data analytics & decision support.
I suspect what’s preventing people from entering the space is an assumption that data analytics use cases are too heterogeneous: every company has its own data with its own peculiarities, answering its own business questions.
But, when I look at the projects themselves, they typically cluster into two use cases:
- Pulling structured information out of unstructured information. Some examples include:
- Bank of England is using LLMs to process 1.5TB of trade data (job post for this use case)
- Astrazeneca wants to analyze internal unstructured documents from their content management system to structure results from their Extractables & Leachables testing (job post)
- Klue, a competitive intelligence product, uses GenAI to draw competitive intelligence insights from sources such as news, competitor website changes, call transcripts and customer reviews (job post)
- Automating actual analysis that was previously done manually:
- Walmart’s Market Intelligence Team is using LLMs to analyze data to inform product selection and pricing decisions (job post)
- Trafigura wants to analyze internal documents to generate credit reports for commodity trading use cases (job post)
- NielsenIQ’s Applied Research and Innovation Team is using LLMs to analyze consumer behavior and market trends. (job post).
Another reason this space may have been overlooked is that the startup/VC community believes strong incumbents like Databricks and Snowflake are best placed to capitalize on the opportunity. But that’s not obviously different from the software engineering, sales & marketing verticals and customer support verticals, where companies like Microsoft, Salesforce and ServiceNow are also making large investments.
In a recent episode of the No Prior podcast, OpenAI Chairman Bret Taylor pointed to ”automating the role of an analyst” as the most promising opportunity for the next wave of GenAI startups. The data in this post suggests he might be right.
Note: you can interact with the data powering this post on Marimo